-
- Mod 5 - Ord Reg
- 5.1 Introduction
- 5.2 Ordinal Outcomes
- 5.3 Assumptions
- 5.4 Example 1 - Ordinal Regression on SPSS
- 5.5 Teacher Expectations and Tiering
- 5.6 Example 2 - Ordinal Regression for Tiering
- 5.7 Example 3 - Interaction Effects
- 5.8 Example 4 - Including Prior Attainment
- 5.9 Proportional Odds Assumption
- 5.10 Reporting the Results
- 5.11 Conclusions
- 5.12 Other Categorical Models
- Quiz
- Exercise
- References
- Mod 5 - Ord Reg
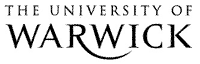
5.12 Other Types of Categorical Models
The PO model used in SPSS is by far the most common form of ordinal regression. However there are other approaches to ordinal regression, for example the continuation ratio model and the adjacent categories model. Both involve restructuring your dataset, sometimes using link functions other than the logit and sometimes statistical packages other than SPSS. They are therefore not core to our coverage in this module. The interested reader can find excellent treatment of such models in O’Connell (2006).
You might also come across a range of models called log-linear models as you read statistics texts (for light reading on a Sunday morning in bed perhaps). This class of models is used where we are interested in the relationships between a set of variables but have no clear outcome or dependent variable. However where we have a variable that clearly represents an outcome (such as a test score, NC level, tier of entry etc.) it makes sense to analyse this in a regression framework asking how various explanatory variables impact on the outcome variable. In such cases a linear, logistic or ordinal regression model is more appropriate than log-linear analysis. For an introduction to log-linear analysis including a guide to implementation in SPSS we recommend Acton & Miller (2009) Chapter 11.
Another type of model is multinomial logistic regression. In such models there is a clear outcome variable, but there is usually no ordinality in the outcome. An example in education might be in identifying the characteristics of students who select different kinds of secondary schools (e.g. Independent schools, Church schools or Local Authority schools). In this case there are therefore multiple outcomes but no ordinality in the outcome. Some authors recommend undertaking multinomial regression even with an ordinal outcome if the PO assumption is not met (e.g. Norusis, 2005). However we can see no advantage to this, indeed if there is ordinality in your outcome and the PO assumption is not supported it is definitely better to complete a series of logistic regressions on cumulative splits in the outcome as demonstrated in this module. For good coverage on multinomial logistic regression we recommend Field (2009) Chapter 8. |